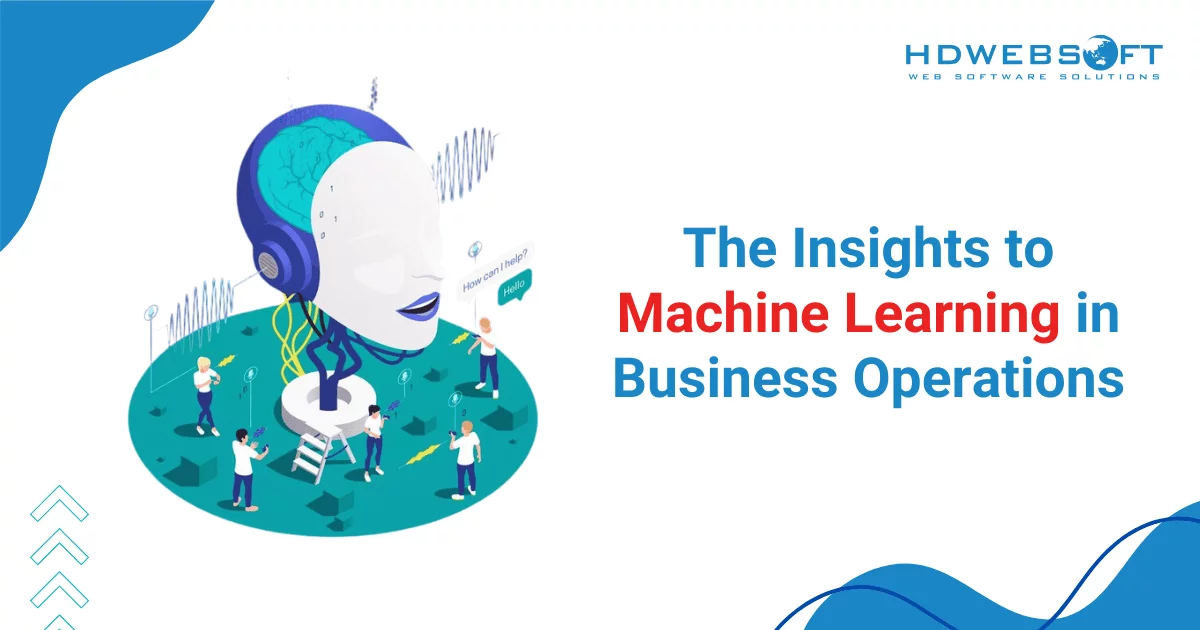
The Insights to Machine Learning in Business Operations
In recent years, machine learning in business has shifted from an experimental concept to a transformative force of innovation. Businesses today navigate vast data landscapes where strategic ML streamlines operations and enables personalization, predictive analytics, and automation. According to recent research, 63% of companies have planned to maintain or boost their AI and ML budgets. This statistic underlines the rising importance of ML in achieving competitive advantages.
In today’s writing, we will dive into the application and potential of machine learning in business operations. But first, we’ll help you understand its foundation and examples of different ways companies can benefit from it.
The Concept of Machine Learning
Machine learning is a branch of artificial intelligence that enables systems to learn from data and identify patterns automatically. It allows machines to make predictions or decisions without explicit programming. In contrast to traditional software, where outcomes follow pre-defined rules, ML models improve over time. They process new data and adjust their parameters for greater accuracy.
There are different types of machine learning methods, each valuable in various business scenarios. These methods enable organizations to tackle unique challenges by selecting approaches that best fit their needs. With an appropriate machine learning model at hand, businesses can respond to changing conditions and maintain a competitive edge in dynamic markets.
In machine learning in business, systems like chatbots, fraud detection tools, and recommendation engines provide real-time insights and automated responses. This not only enhances customer experience but also increases operational efficiency.
Overall, the ability of ML to automate with minimal human intervention makes it a powerful tool in the business landscape.
Different Types of Machine Learning in Business Operations
Machine learning algorithms come in various forms, each designed for specific types of data and goals. Each algorithm offers unique ways to handle data, automate processes, and deliver actionable insights. Here’s a breakdown of the main types of machine learning algorithms and how they contribute to business innovation.
Supervised Learning
Supervised learning is one of the most commonly used methods of machine learning in business. It involves training a model on labeled data, where both the input and desired output are known. Through this approach, the model learns to associate inputs with outputs and can make predictions on new data.
In business, supervised learning is used for tasks like customer segmentation, sales forecasting, and spam detection. For example, banks often use it to assess credit risk by training models on historical data that include customer details and loan repayment records. This banking AI helps them to predict the likelihood of future clients defaulting, which helps manage risk and improve decision-making.
Supervised machine learning in business compares its predictions against the correct labels and adjusts its model accordingly.
Unsupervised Learning
On the contrary, unsupervised learning deals with unlabeled data. It means that the algorithm has to find patterns and relationships without predefined outcomes. Owing to that, this type of machine learning is ideal for clustering and association tasks.
In the context of business, unsupervised learning is especially valuable for uncovering hidden patterns in customer behavior. For instance, AI e-commerce platforms use clustering algorithms to group customers with similar purchasing behaviors, helping marketers create targeted campaigns.
Additionally, this type of machine learning in business can be applied in anomaly detection, where it helps identify unusual transactions. This may indicate fraud in financial services.
Semi-supervised Learning
This is a hybrid version falling between supervised and unsupervised learning. The models are trained on a mix of labeled and unlabeled data, enabling them to recognize patterns and make predictions based on these learned insights. In particular this method is especially useful when labeling data is costly or time-consuming.
Semi-supervised learning is commonly applied in scenarios like image recognition and document classification. To illustrate, let’s say a retailer with a large number of product images may have only a few labeled samples. By using this ML technology, the algorithm can leverage both labeled and unlabeled data to classify products accurately. As a result, it can enhance inventory management and improve the online shopping experience.
Reinforcement Learning
This is another area of machine learning in business where an algorithm learns by interacting with an environment and receiving feedback in the form of rewards or penalties. This method is valuable for tasks that require sequential decision-making, such as robotics, gaming, and process automation.
For starters, reinforcement learning is often used to optimize logistics and supply chain management. Namely, a delivery service might use reinforcement learning to optimize delivery routes, reduce costs, and improve delivery times. By constantly learning and adjusting based on results, these algorithms help businesses improve efficiency and adapt to changing conditions.
Transfer Learning
Another well-known technique in the world of machine learning is transfer learning. In this approach, the model is initially trained on one task and then adapted for a different, related task. Consequently, this method becomes highly useful when there is limited data available for a specific business problem,
This ML approach can save time and resources by leveraging previously gained knowledge in similar domains. In machine learning in business, transfer learning is used for tasks like natural language processing (NLP) and image recognition. For instance, a company developing a new product categorization tool can utilize a pre-trained model. This model has already learned to identify general categories, thereby speeding up the deployment of the new system.
Federated Learning
This is a newer machine learning technique – also referred to as collaborative learning. The model is trained across multiple decentralized devices or servers without transferring the data to a central location. This method prioritizes privacy and data security, as the data remains on local devices, and only model updates are shared.
Machine learning in business often involves sensitive customer data, so federated learning is beneficial in sectors like healthcare and finance. For example, healthcare organizations can use federated learning to develop predictive models for patient outcomes across various facilities without centralizing patient data. Consequently, this approach ensures compliance with privacy regulations while allowing for the sharing of collective insights to improve healthcare services.
Machine Learning Applications in Business
ML capabilities are not limited to a specific industry or sector or restricted to a single function or task. Here are some of the primary ways machine learning is applied in the business world today.
Text Parsing
Can machine learning help narrow the communication gap between technology and humans? Perhaps, but one certainty is that NLP, a branch of ML, can now understand, analyze, and process text created by humans. As a result, this technology enables it to derive meaning from human language.
For machine learning in business, text parsing is commonly used for sentiment analysis. This is where companies scan customer reviews and social media posts to gauge customer satisfaction. Desirably, businesses are provided with valuable insights into brand perception, enabling them to address customer concerns more proactively.
Additionally, text parsing powers content filtering and spam detection. They are especially necessary for managing communications and preventing harmful content in real time.
Image Recognition and Classification
ML models can interpret and analyze not only text but also visual data, thereby identifying patterns, objects, and scenes within images. This powerful capability falls under the field of computer vision. As a result, image recognition and classification are revolutionizing various sectors, particularly in retail and manufacturing.
This machine learning in business operations is integrated in many aspects of life, more than you think!
Specifically, online retailers use image recognition to allow customers to search for products based on pictures. It will help improve the shopping experience and make product discovery easier. Meanwhile, manufacturing industries apply image classification to monitor product quality, detect defects early in the production line, and reduce waste.
As can be seen, the above examples have demonstrated the power of machine learning in business in many ways possible.
Fraud Detection
Fraudsters are becoming more sophisticated daily, making it difficult for financial institutions and other businesses to identify fraudulent activities consistently. As online transactions increase, traditional methods for detecting fraud have grown less effective.
Thankfully, machine learning offers valuable assistance in creating more effective solutions to this issue. Analyzing vast amounts of data in real-time and using advanced algorithms can identify critical patterns and detect anomalies. Whether dealing with insurance fraud, credit card fraud, or suspicious transactions, ML models can learn from historical data and flag any unusual activity that may indicate potential fraud.
According to a report, machine learning-driven fraud detection systems have achieved 96% accuracy in reducing fraud for eCommerce businesses. Therefore, machine learning technology has demonstrated its value in combating fraud, with the potential to save millions in losses.
Customer Service (Chatbots & NLP)
Machine learning in business is transforming customer service through chatbots, voice chatbots, and natural language processing. With this technology, chatbots powered by machine learning can handle customer queries 24/7. Consequently, they provide immediate responses to routine questions, allowing human agents to focus on more complex issues.
Chatbots learn to handle customer queries using machine learning in business systems.
Moreover, NLP enables chatbots to understand customer language and intent, making interactions feel more natural and responsive. These virtual agents not only improve response times but also analyze previous interactions to offer personalized support. Ideally, they can become a massive boost to customer satisfaction and loyalty.
Recommendation Engines
The ML algorithm has become a critical component of recommendation engines. They assist businesses in predicting customer demand and customizing user experiences. Ever wondered how platforms like Netflix, Amazon, or YouTube suggest content or products? It’s all thanks to machine learning algorithms.
By analyzing existing data—such as user behavior and preferences—these engines can uncover trends and generate personalized suggestions. This process results in more engaging, tailored recommendations for end users, which in turn boosts customer retention and drives sales.
To demonstrate how impactful these engines can be in machine learning in business, we’ll give an example. According to the business itself, Netflix’s recommendation engine accounts for over 80% of the content watched on the platform.
Customer Churn Modeling and Segmentation
As one of the longstanding challenges in business, customer churn can be reduced with the help of machine learning solutions. Typically, customer churn leads to significant losses when clients leave for competitors or cancel their subscriptions.
In this context, machine learning algorithms analyze purchase history, engagement frequency, and customer satisfaction scores to predict churn likelihood. Subsequently, businesses can use these insights to create targeted retention campaigns.
Customer churn is no longer a pain point now with the help of machine learning in business.
In short, proactively addressing customer needs and delivering personalized experiences is an effective strategy. With that in mind, machine learning in business enables companies to maintain stronger relationships and foster customer loyalty.
Real-life Examples of Machine Learning in Business
Machine learning has proven its transformative potential across various industries, improving processes, customer experiences, and decision-making. Let’s take a look at some real-life examples of how companies are leveraging machine learning to drive tangible results.
- Amazon’s Recommendation System: Amazon’s use of machine learning for its recommendation engine has set an industry benchmark. The system analyzes browsing history, previous purchases, and customer interactions to provide personalized recommendations, increasing both sales and customer satisfaction.
- Coca-Cola’s Supply Chain Management: Coca-Cola uses ML algorithms to manage its supply chain. The company analyzes data from vending machines and retail channels to ensure products are available where and when needed. Ultimately, their decision has optimized logistics and cut costs for the business as a whole.
- Uber’s Surge Pricing: Uber employs machine learning in business to adjust pricing in real-time, responding to demand changes in different areas. The system takes data like traffic, rider demand, and time of day into account. As a result, it creates dynamic pricing that benefits both drivers and passengers.
- Spotify’s Personalized Playlists: Spotify leverages ML to curate personalized playlists for each user, enhancing the listening experience. By analyzing user preferences, listening history, and contextual data, Spotify keeps users engaged with tailored recommendations.
Final Note
The growing influence of machine learning in business is undeniable. Eventually, machine learning and AI will make their mark in nearly every aspect of our lives, be it business or daily life. Ongoing advancements and innovations in this area mean we can anticipate even more impressive developments from ML models.
Given this, businesses should begin investing in machine learning technology to remain relevant in today’s fast-paced environment. It’s essential to have a partner equipped to help businesses like yours set clear goals and embark on the AI journey toward success. There is no need to consider any longer, we’re here to help.
With HDWEBSOFT’s AI development services, we’ve helped countless businesses of all sizes and industries turn their ideas into advanced software solutions. Get to know HDWEBSOFT and talk to our experts today.