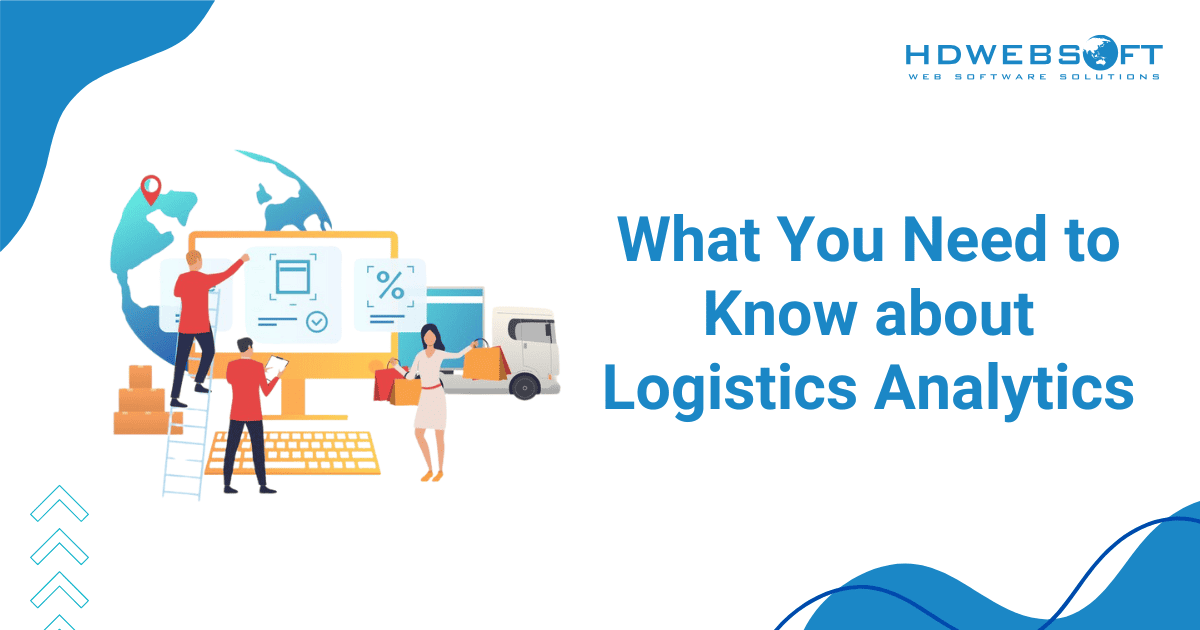
Logistics Analytics 101: What You Need to Know
Logistics analytics is essential in today’s fast-paced world, where businesses depend on efficient, responsive supply chains to stay competitive. This field leverages data and advanced analytical tools to optimize the complex logistics processes that connect products to customers. As global trade expands, logistics analytics will be increasingly crucial for businesses looking to streamline operations and enhance customer satisfaction.
In today’s article, we’ll go through the definition of logistics analytics, along with its type and benefits. Moreover, we’ll provide actionable insights from real-life examples and how HDWEBSOFT can help you implement the technology.
What is Logistics Analytics?
Logistics analytics leverages data, algorithms, and machine learning to enhance logistics operations by analyzing vast datasets across the supply chain. The amount of data accessible for logistics analytics is growing swiftly as digitalization progresses across industries.
Recent reports also reveal notable increases in both the size of this data and investment in analytics-driven logistics solutions. For instance, the industry has witnessed over 31,000 new patents filed, signaling strong innovation and financial interest. Additionally, the workforce has grown by 796,000 employees globally, reaching a total of 14.5 million workers. This statistic has further highlighted the sector’s critical role in global operations and resilience strategies.
When applying these logistics analytics tools, companies can identify patterns, predict demand, and simulate scenarios to make informed, adaptive decisions. Particularly, this adaptability is valuable in anticipating and responding to potential disruptions. Furthermore, analytics-driven insights provide a competitive edge by enabling cost reductions and improving service levels. They also drive operational efficiencies and allow businesses to tackle challenges proactively in real-time.
Types of Logistics Analytics
In the evolving field of logistics, businesses are harnessing data-driven insights for multiple purposes. By leveraging different types of analytics, companies can explore past patterns, understand present inefficiencies, and prepare for future demands. Here’s a closer look at the five key types of logistics analytics: Descriptive, Diagnostic, Predictive, Prescriptive, and Cognitive Analytics.
Descriptive Analytics
Descriptive analytics serves as the foundation for understanding the current state of logistics. By analyzing historical data, it answers basic questions like “What happened?” and “Where are the inefficiencies?” This approach gives companies a clear picture of their past performance and current trends, which is essential for spotting patterns.
For starters, descriptive analytics in logistics can provide insights into delivery times, order volumes, warehouse efficiency, and fuel consumption. These insights will help teams understand baseline performance. For example, by reviewing monthly delivery data, companies might identify seasonal demand spikes and plan better for peak periods. Descriptive analytics, while retrospective, forms a crucial basis for more advanced analytics.
Diagnostic Analytics
While descriptive analytics tells us what happened, diagnostic analytics goes deeper, asking, “Why did this happen?” This layer of logistics analytics is about investigating the root causes behind trends and outcomes. If a certain transportation route experiences recurring delays, diagnostic analytics would examine factors like traffic patterns and weather conditions. In addition, it would assess fleet efficiency to identify the underlying reasons behind these delays.
Another thing is that by pinpointing specific causes, companies can address problems directly and avoid similar disruptions in the future. Diagnostic analytics often involves data visualization tools that make it easier to spot outliers and patterns. Ultimately, it’s highly actionable for logistics managers looking to solve inefficiencies.
This type of logistics analytics will get to the roots and address problems.
Predictive Analytics
Using historical data and machine learning prediction models, predictive analytics enables businesses to forecast future outcomes. This type of analysis answers the question, “What is likely to happen next?”
For instance, predictive models can anticipate demand fluctuations, helping companies adjust inventory levels and prepare for peak periods. By understanding future demand, businesses can allocate resources more effectively, avoid stockouts, and improve delivery timelines. This is especially useful for e-commerce businesses that leverage AI for their operations.
Additionally, predictive logistics analytics aids in route optimization by forecasting traffic conditions and estimating the best times for delivery. With predictive insights, logistics managers can stay one step ahead, reducing costs and improving customer satisfaction by meeting demands promptly.
Prescriptive Analytics
Building on predictive insights, prescriptive analytics provides actionable recommendations on “What should we do?” It uses algorithms and optimization models to suggest the best course of action based on predicted scenarios.
For example, prescriptive analytics in logistics could recommend the most efficient transportation routes. It might also suggest optimal inventory levels or propose adjustments to staffing in the warehouse based on anticipated demand.
By guiding decision-making, the technology allows companies to make proactive, data-backed adjustments that minimize risk and maximize efficiency. Consequently, this type of analytics is highly valuable for complex logistics operations where there are multiple variables and potential solutions.
Cognitive Analytics
Cognitive analytics represents the cutting edge of logistics analytics, utilizing artificial intelligence and machine learning for business operations. These technologies process and interpret vast amounts of both structured and unstructured data, enabling deeper insights into logistics operations. What’s more, it attempts to mimic human thought processes, making sense of data and other qualitative information.
Likewise, the technologies help companies address nuanced issues, like understanding customer sentiment and predicting potential service issues before they escalate. By integrating insights from these varied sources, cognitive analytics supports highly personalized customer service and smarter decision-making. Namely, a logistics company might use cognitive analytics to analyze customer reviews and adjust delivery practices to improve satisfaction.
Logistics analytics will assist in analyzing customer sentiments, thus helping businesses enhance customer satisfaction.
Benefits of Big Data Analytics in Logistics
The rise of analytics-driven logistics through big data has transformed the industry. Let’s explore some of the most impactful benefits that big data analytics brings to logistics.
Enhanced Operational Efficiency
One of the key benefits of logistics analytics is improved operational efficiency. By analyzing data from various sources—such as GPS, RFID, sensors, and more—logistics companies can optimize their daily operations.
For example, data analysis can reveal optimal delivery routes based on historical traffic patterns, helping drivers avoid congested areas and minimize delivery times. This reduces fuel consumption, lowers costs, and increases the number of deliveries that can be completed in a day. Additionally, data-driven insights allow companies to better manage warehouse operations by organizing inventory more effectively and reducing time spent locating and handling goods.
Better Demand Forecasting
As a core component of big data, predictive analytics help logistics companies anticipate demand more accurately. By examining historical data, seasonal patterns, and market trends, companies can better forecast the required goods volume at specific times. This is especially valuable for managing inventory and avoiding stockouts or overstocking situations, which can disrupt the supply chain.
For example, during peak seasons, demand forecasting can help companies stock up adequately to meet customer needs without creating excessive inventory costs. According to a report, predictive logistics analytics has improved demand forecast accuracy in supply chains by up to 20%. Through this statistic, we can see that the technology translates to more effective planning and resource allocation.
Cost Reduction
Analytics in logistics provides companies with a clear understanding of their cost structure. Desirably, they can pinpoint areas where expenses can be cut without sacrificing quality or efficiency. By analyzing fuel consumption, labor costs, and vehicle maintenance needs, companies can implement strategies to reduce these expenses.
For instance, big data and analytics can suggest optimized routes and delivery schedules, cutting down fuel usage and overtime costs. Additionally, predictive maintenance powered by analytics can help reduce vehicle breakdowns. They assist in minimizing repair costs and avoiding unexpected disruptions in delivery schedules.
Reducing unwanted costs is one of the benefits of logistics analytics.
Improved Customer Satisfaction
With the help of logistics analytics, companies can offer enhanced services that boost customer satisfaction. Real-time tracking, for example, allows customers to follow their shipments and receive accurate delivery time estimates. This transparency is highly valued by customers and can be a deciding factor when choosing a logistics provider.
On top of that, with the technology at hand, companies can personalize their services and offer tailored solutions that meet specific needs. Higher customer satisfaction not only strengthens brand loyalty but can also lead to increased referrals and a stronger market reputation.
Risk Mitigation
Big data analytics helps logistics companies anticipate and manage potential risks. For instance, data-driven models can identify weather patterns, geopolitical risks, and supply chain disruptions. By then, companies can adjust routes or schedules to avoid delays.
As a result, this proactive logistics analytics approach helps minimize risks associated with operations. In addition, it’ll ensure that companies can continue delivering products on time even in unpredictable situations. Moreover, logistics companies can analyze previous incidents to understand root causes, helping them implement better preventive measures in the future.
Sustainability and Environmental Impact
With increased awareness of sustainability, logistics companies are under pressure to reduce their environmental impact. Big data analytics can play a significant role here by helping companies track and optimize their carbon footprint.
To illustrate, companies can analyze fuel consumption and emissions to implement eco-friendly practices. This might involve consolidating deliveries to reduce trips or using optimized routes that minimize fuel use. Resultantly, these sustainable practices help the environment and improve a company’s public image, appealing to environmentally conscious consumers and partners.
Further reading: Logistics Automation in Modern Supply Chain.
Actionable Insights from Logistics Analytics Examples
Data analytics provides a wealth of actionable insights to help companies optimize their supply chains, improve efficiency, and boost profitability. Let’s take a look at some key examples of how logistics analytics delivers actionable insights.
Inventory Optimization
Logistics analytics allows companies to achieve a delicate balance in inventory management by analyzing demand patterns, seasonal trends, and replenishment cycles. With inventory optimization, businesses can ensure they have the right stock levels, reducing the risk of stockouts or overstock situations.
Specifically, analytics can help a retail company predict high-demand periods, allowing it to stock up accordingly. They’ll help reduce costly delays and enhance customer satisfaction. Furthermore, optimization helps keep storage costs low while ensuring that goods are available when needed.
Warehouse Slotting
Warehouse slotting is the process of organizing products within a warehouse to maximize space and streamline picking and packing times. Through analytics, companies can analyze historical order data to identify which products are frequently ordered together or require rapid dispatch. By placing high-demand items in easily accessible locations, companies can reduce order fulfillment time and labor costs. Ultimately, the overall efficiency will be improved.
This is all about putting the stocks at the right place to be used when the right time comes.
Particularly, this insight is beneficial for e-commerce businesses, where quick and accurate delivery is essential for customer satisfaction.
Supplier Evaluation
Evaluating suppliers is essential for maintaining a smooth, reliable supply chain, and logistics analytics can play a critical role here. Supplier performance data, such as on-time delivery rates, quality of goods, and cost efficiency, will be analyzed. Once it’s done, businesses can assess which suppliers consistently meet their standards and which may need improvement.
Ideally, logistics teams can make strategic sourcing decisions and work only with suppliers who align with their goals. As a consequence, it’ll reduce disruptions and maintain a higher level of product quality for customers.
Workforce Productivity Analysis
Workforce productivity is another area where analytics for logistics provides valuable insights. Analytics can suggest optimal staffing levels for various shifts. This ensures sufficient workforce coverage during high-demand times while avoiding overstaffing during quieter periods. As a result, companies can enhance productivity, reduce labor costs, and improve job satisfaction among employees.
Market Penetration
Last but not least, logistics analytics enables companies to gain insights into new market opportunities. By examining data on regional demand, customer demographics, and competitive activity, companies can identify areas with high potential for market expansion. Consequently, this insight lets them allocate resources effectively, focusing on regions where demand for their products is highest.
Namely, a logistics firm could analyze shipment data to identify cities with high shipping volumes for a specific product category. Based on these insights, the firm could target those areas for new facilities or optimize delivery routes to improve efficiency.
Further reading: An Ultimate Guide in Machine Learning in Marketing.
How HDWEBSOFT helps with Data Analytics
At HDWEBSOFT, we understand that adopting logistics analytics can be a complex process involving data integration, technological investment, and specialized skills. That’s why we offer end-to-end support to help companies embrace analytics-driven logistics strategies.
- Custom Analytics Solutions: We design and implement analytics systems tailored to your unique business needs. We’ll make sure that you gain actionable insights that drive real impact.
- Data Integration and Management: Our team can assist in collecting and integrating data from various sources, making it accessible for analysis. Your data will be clean, secure, and structured for optimal analysis.
- Training and Support: Training is on us, we’ll equip them with the knowledge and tools to use logistics analytics effectively. Our support team is always on hand to assist with troubleshooting and optimization.
- Ongoing Optimization: Our solutions are designed to adapt as your business grows. We work with you to continuously enhance and refine your logistics analytics strategy, ensuring you remain competitive and resilient.
With HDWEBSOFT as your partner, you’ll be prepared to leverage big data & analytics to its full potential. Let us empower your business to make data-driven decisions that drive success in a dynamic logistics application development market.